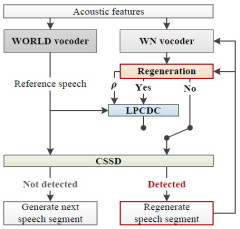
Non-parallel Voice Conversion System with WaveNet Vocoder and Collapsed Speech Suppression
In this paper, we integrate a simple non-parallel voice conversion (VC) system with a WaveNet (WN) vocoder and a proposed collapsed speech suppression technique. The effectiveness of WN as a vocoder for generating high-fidelity speech waveforms on the basis of acoustic features has been confirmed in recent works. However, when combining the WN vocoder with a VC system, the distorted acoustic features, acoustic and temporal mismatches, and exposure bias usually lead to significant speech quality degradation, making WN generate some very noisy speech segments called collapsed speech. To tackle t...