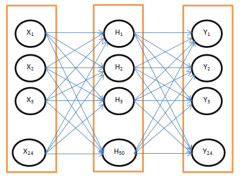
Vowels and Prosody Contribution in Neural Network Based Voice Conversion Algorithm with Noisy Training Data
This research presents a neural network based voice conversion (VC) model. While it is a known fact that voiced sounds and prosody are the most important component of the voice conversion framework, what is not known is their objective contributions particularly in a noisy and uncontrolled environment. This model uses a 2-layer feedforward neural network to map the Linear prediction analysis coefficients of a source speaker to the acoustic vector space of the target speaker with a view to objectively determine the contributions of the voiced, unvoiced and supra-segmental components of sounds t...