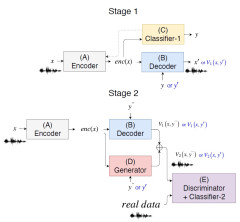
Multi-target Voice Conversion without Parallel Data by Adversarially Learning Disentangled Audio Representations
Recently, cycle-consistent adversarial network (Cycle-GAN) has been successfully applied to voice conversion to a different speaker without parallel data, although in those approaches an individual model is needed for each target speaker. In this paper, we propose an adversarial learning framework for voice conversion, with which a single model can be trained to convert the voice to many different speakers, all without parallel data, by separating the speaker characteristics from the linguistic content in speech signals. An autoencoder is first trained to extract speaker-independent latent rep...