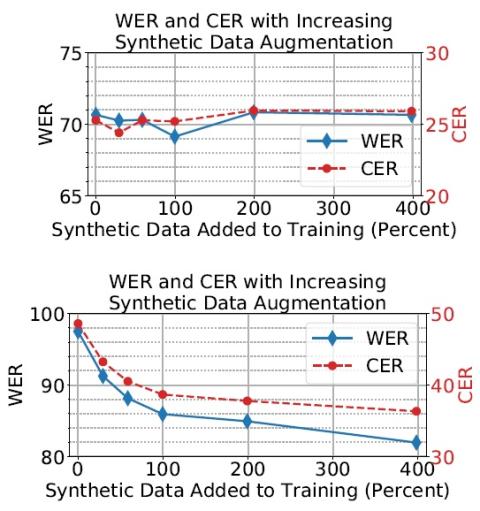
Measuring the Effectiveness of Voice Conversion on Speaker Identification and Automatic Speech Recognition Systems
This paper evaluates the effectiveness of a Cycle-GAN based voice converter (VC) on four speaker identification (SID) systems and an automated speech recognition (ASR) system for various purposes. Audio samples converted by the VC model are classified by the SID systems as the intended target at up to 46% top-1 accuracy among more than 250 speakers. This encouraging result in imitating the target styles led us to investigate if converted (synthetic) samples can be used to improve ASR training. Unfortunately, adding synthetic data to the ASR training set only marginally improves word and charac...