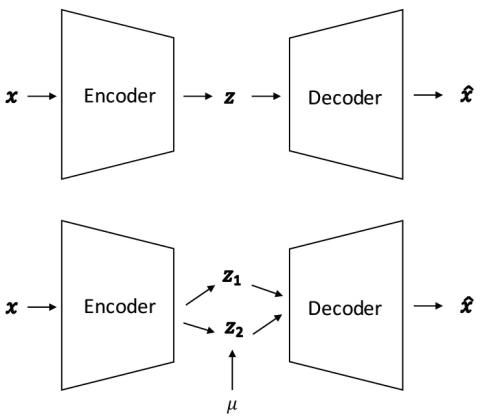
Investigation of Using Disentangled and Interpretable Representations for One-shot Cross-lingual Voice Conversion
We study the problem of cross-lingual voice conversion in non-parallel speech corpora and one-shot learning setting. Most prior work require either parallel speech corpora or enough amount of training data from a target speaker. However, we convert an arbitrary sentences of an arbitrary source speaker to target speaker's given only one target speaker training utterance. To achieve this, we formulate the problem as learning disentangled speaker-specific and context-specific representations and follow the idea of [1] which uses Factorized Hierarchical Variational Autoencoder (FHVAE). After train...